HaptX Deep
- Contact:
- Partner:
KIT: Institute for Material Handling and Logistics (IFL, Jun.-Prof. Rayyes)
- Startdate:
01.01.2024
- Enddate:
31.12.2024
Deep learning system for flexible, versatile material handling and manufacturing for the future of innovation
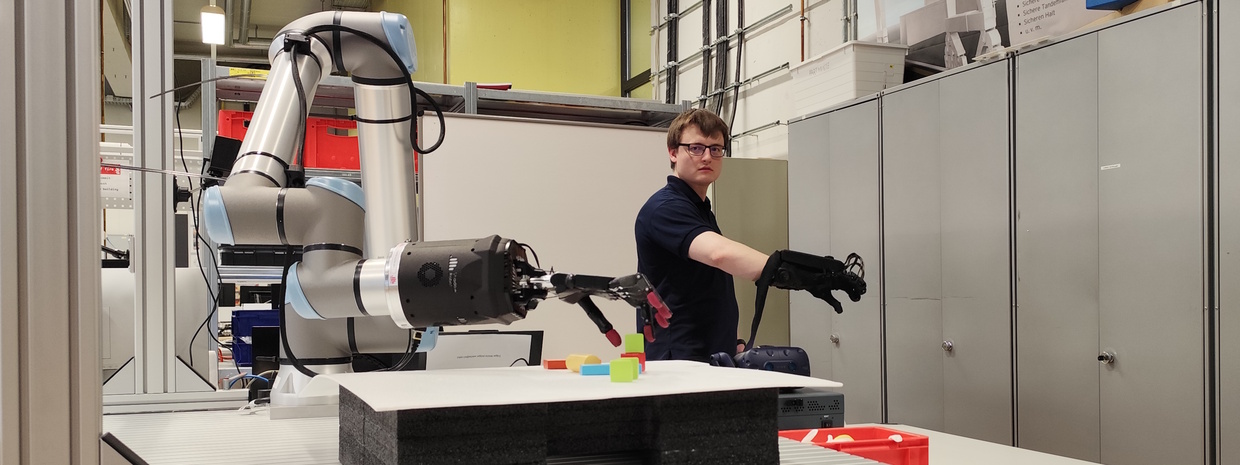
Motivation
Robotic systems for innovative manufacturing must be able to adapt quickly and flexibly to any changes in production requirements, product designs, materials, and suppliers, without the need for reprogramming. The advent of robot learning broadens the scope of potential applications that were previously beyond the capabilities of conventional automation techniques.
However, robots face the challenge of shortening the extensive learning phase while ensuring safety and reliability in industrial environments. Hence, enabling robots to learn human-like skills using innovative technology can accelerate adaptability to new manufacturing tasks and production processes, as well as ensure safety.
Goal
The aim of this project is to develop a robotic gripping system based on the Shadow Robot teleoperation system that can learn human-like skills. The rapid development of new technologies has led to the continuous development of a new generation of industrial products and tools. The adaptation of the robot to handle new objects more efficiently can therefore be achieved by transferring human-like skills into its learning process. Another focus of the project is the consistent evaluation of the safety and reliability of these adaptable robots. Proactive error prediction methods are used to minimize the risk of dangerous events.
Benefit
Changeover time to new components is reduced as the robot adapts itself or with minimal human assistance. The robot's remote guidance enables it to perform tasks in safety-critical areas.